DeepFaceLive is a real time face replacement software, one click installation, newbie friendly, and you can’t see any mistakes after face replacement.
Demo
Welcome to “DeepFace Live”!
No need for plastic surgery! No minimally invasive!
Double eyelid, eye opening, face slimming are not a problem!
You will become a handsome man and a beautiful woman in seconds!
Let me show you the very mature “plastic surgery technology”.
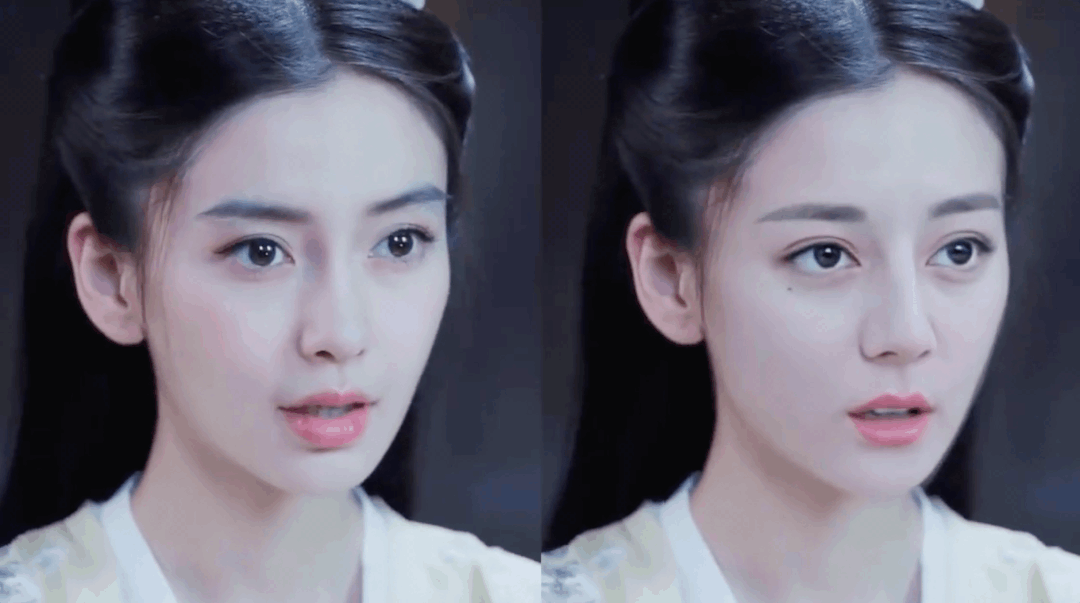
A software can directly transform Angela Baby into Dilraba!
Just want Dilraba’s teardrop and mouth? No problem!
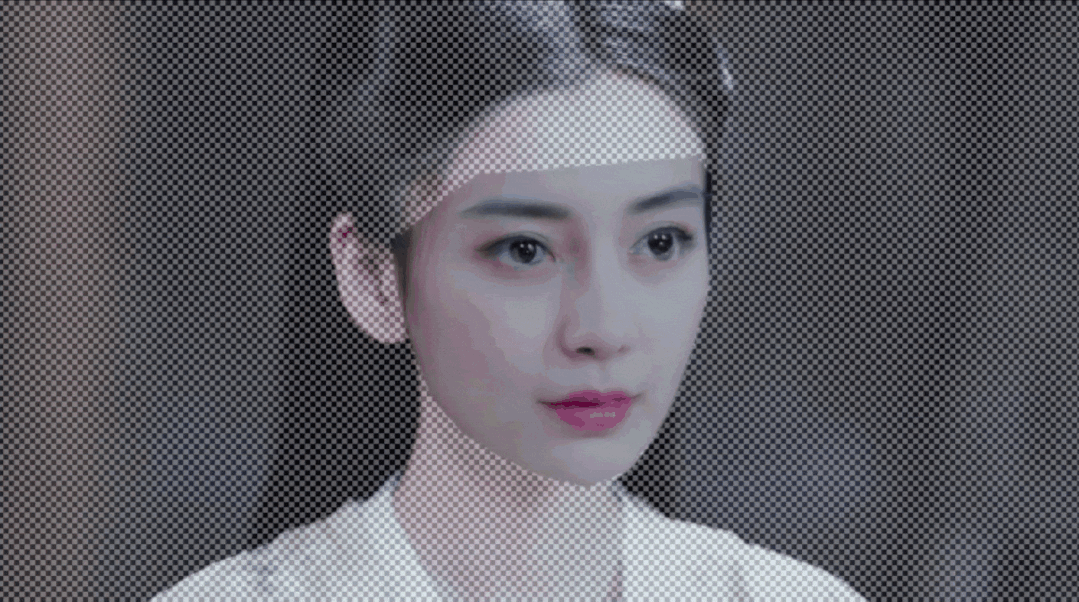
You can also change the face of a blessed comedian Shen Teng into that of Jack Ma.
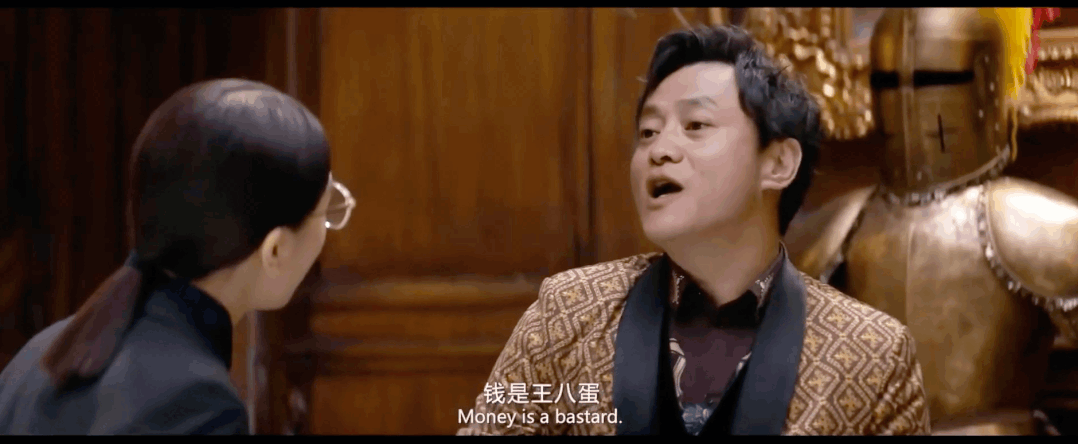
And this time, the “plastic surgery technology” has a new upgrade!
Real-time facelift" for live web stars!
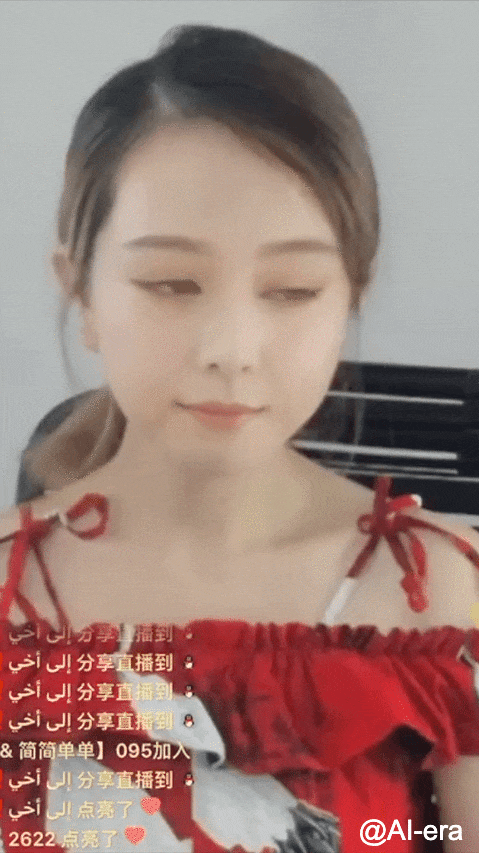
The face of an internet celebrity is replaced with the face of Fan Bingbing, without any sense of “faking”.
That’s right, this is DeepFaceLive’s real-time face changing software.
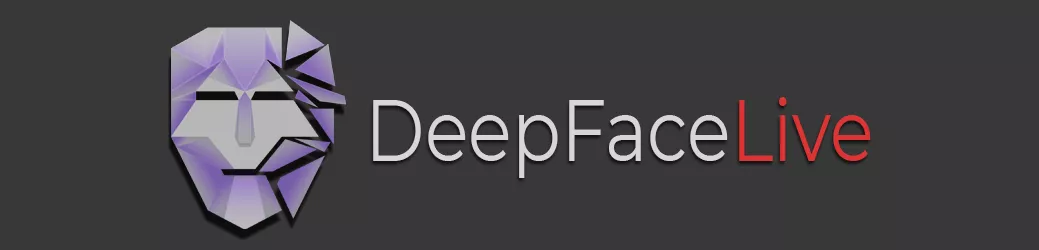
DeepFaceLive project address: https://github.com/iperov/DeepFaceLive
Just open the software, you will be able to process the video in real time and give the live broadcaster a new face.
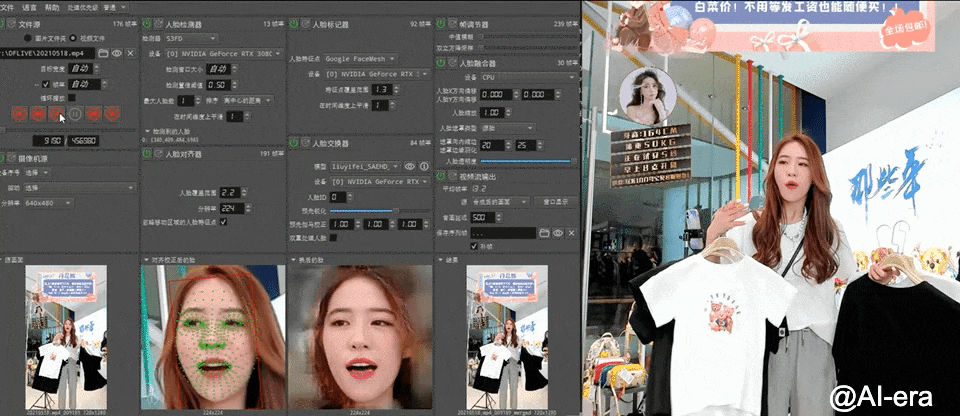
Here is a big weapon: Liu Yifei model!
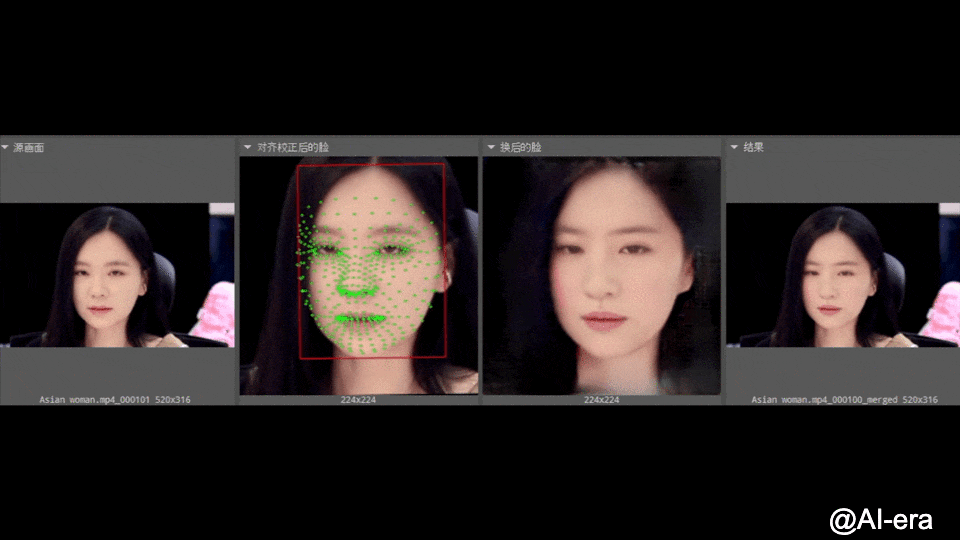
Zoom in to see, the five features are perfectly replaced.
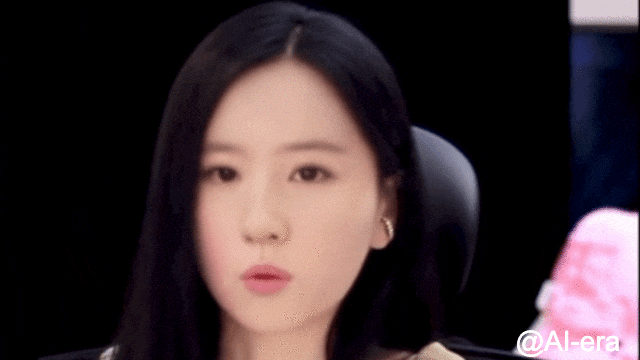
DeepFaceLive is also able to handle different gender face replacement.
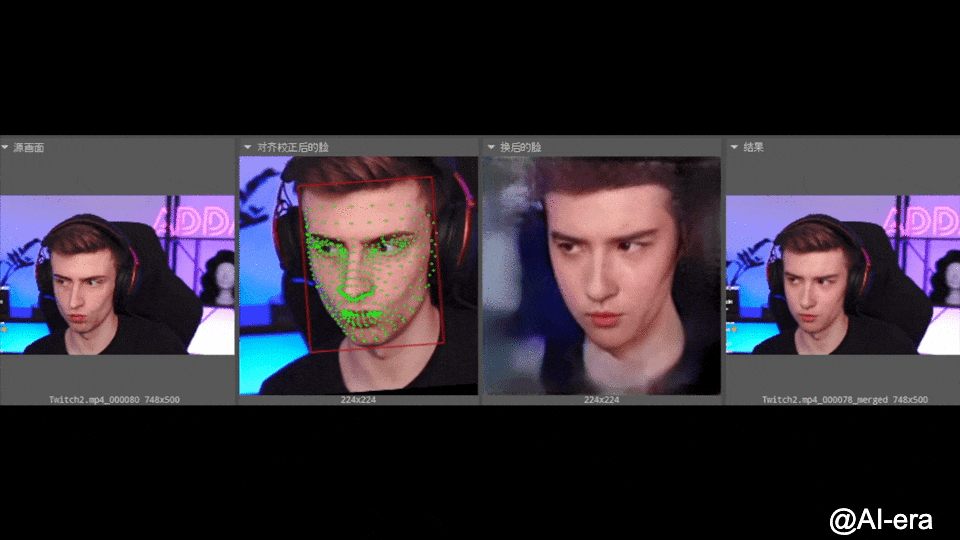
The software launched by the team this time can change faces in real time for live video, but also during video calls.
Behind the Scene
Of course, the model of face replacement has to be trained by DeepFaceLab algorithm first.
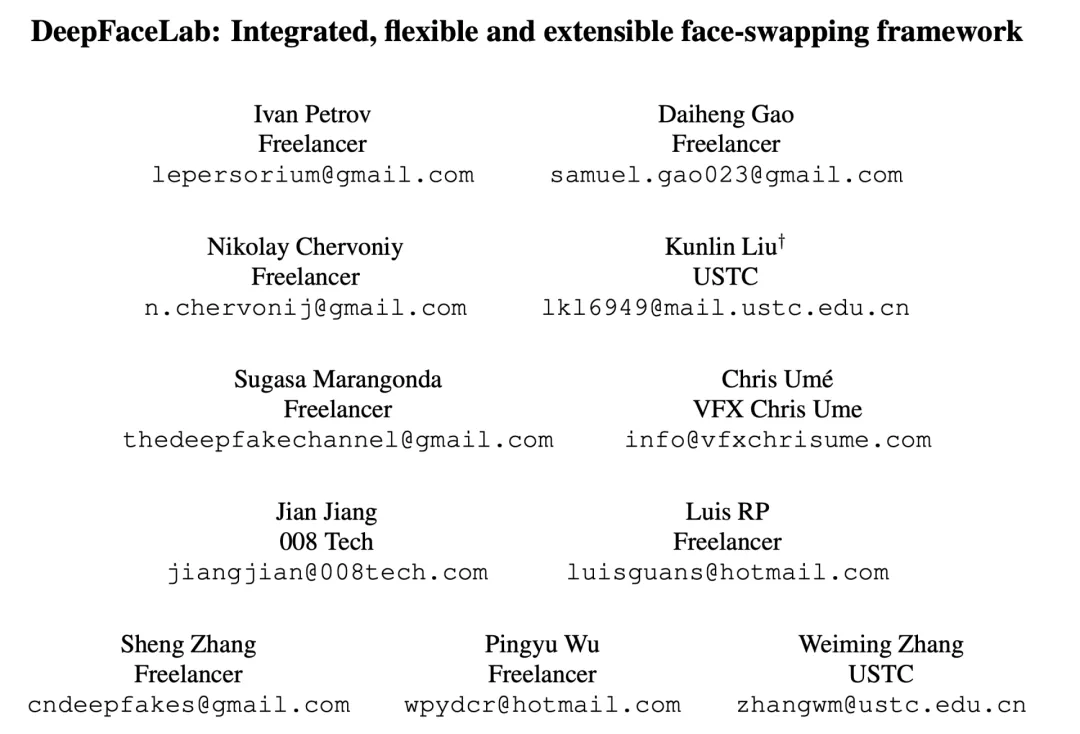
Address: https://arxiv.org/pdf/2005.05535.pdf
More than 95% of the Deep Fake videos on the web are now created with DeepFaceLab.
For example, the following popular YouTube channels.
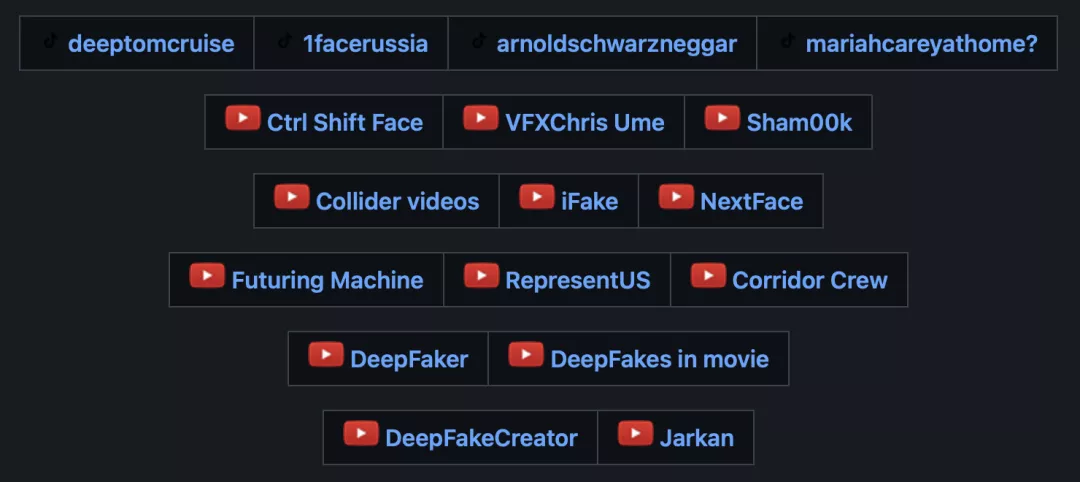
DeepFaceLab project address: https://github.com/iperov/DeepFaceLab
With the launch of DeepFaceLive, there will definitely be more fun videos and even live streams.
The software is also very easy to run, requiring only a 64-bit Win 10 system and an NV graphics card.
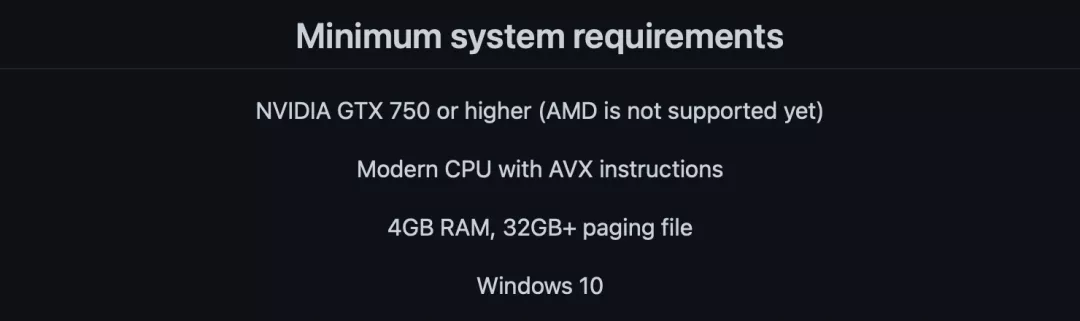
That’s it!
DeepFaceLive
Just click and change!
(Remember to update your graphics card drivers)
Face Detection
The face detector is integrated with YoloV5, S3FD and CenterFace.
You can also choose to use the CPU for processing.
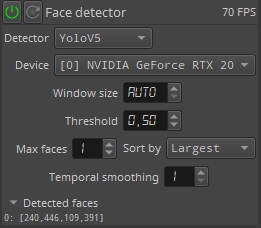
Face Alignment
Simple parameters can be modified to adjust the effect of face alignment.
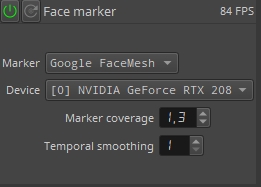
Face Marker
The face tagger provides CPU-based OpenCV LBF and GPU-based Google FaceMesh.
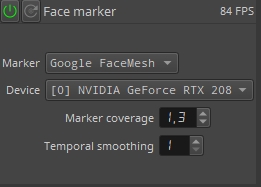
Face Swapper
In the face swapper, you need to load a model trained by the user in advance with DeepFaceLab.
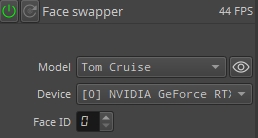
A detailed tutorial can be found at: https://github.com/iperov/DeepFaceLive/blob/master/doc/setup_tutorial_windows/index.md
DeepFaceLab
Lab enables smooth and realistic face swapping without the need to hand-pick features.
Only two videos are needed: the source video (src) and the target video (dst).
Moreover, the two videos do not need to match the same facial expression between them.
Part 1 Extracting faces
The first stage of Lab is to extract faces from src and dst data.
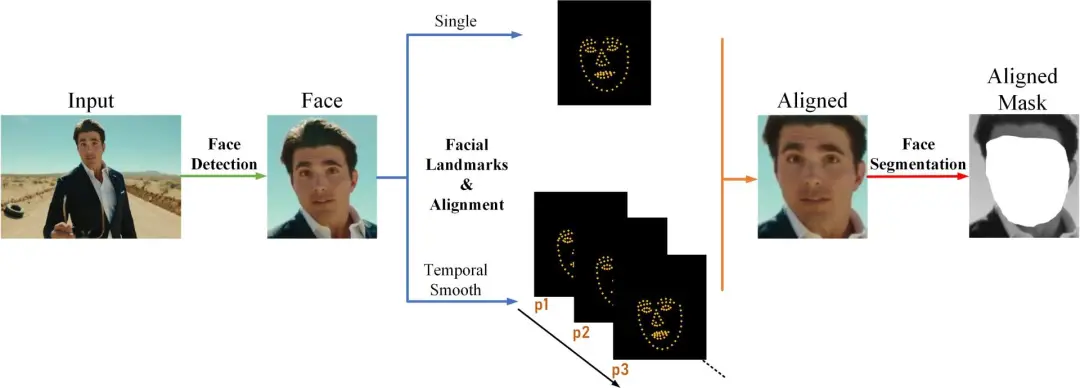
Face Detection
In Lab, S3FD is used as the default face detector.
Face Alignment
Lab provides two typical face coordinate extraction algorithms to solve this problem.
Heat map-based facial coordinate algorithm 2DFAN (for faces with standard pose) PRNet with 3D facial a priori information (for faces with large Euler angles, e.g. one of the sides is out of the line of sight).
After retrieving the face coordinates, Lab provides an optional function with configurable time steps to smooth the face coordinates of consecutive frames in a single shot, further ensuring stability.
The classical point pattern mapping and transformation method is then used to compute the similarity transformation matrix for face alignment.
Since a standard facial coordinate template is required for calculating the similarity transformation matrix, Lab provides a canonical aligned facial coordinate template.
In addition, Lab can automatically predict the Euler angles using the obtained facial coordinates.
Face segmentation
After alignment, a folder of face data with standard front or side views is obtained.
We use a fine-grained face segmentation network (TernausNet) on this basis to accurately segment faces with hair, finger or glasses occlusions, while also removing irregular occlusions.
Since some SOTA face segmentation models are unable to generate fine-grained masks in some specific shots, Lab introduced XSeg into the mix.
XSeg allows users to use multiple photos to train the model to segment specific faces.
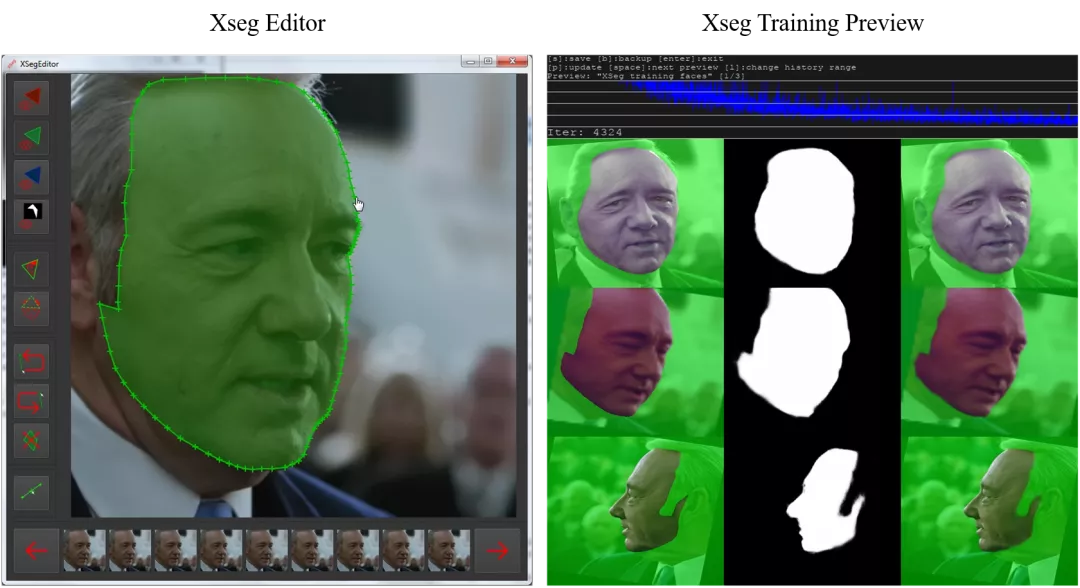
With the help of XSeg, users can use it to remove the occlusion of hands, glasses and any other objects that may cover the face, and control specific areas for swapping.
Part 2 Model training
Since the authors want to avoid a strict matching of src and dst facial expressions, Lab proposes two structures to solve this problem.
DF structure
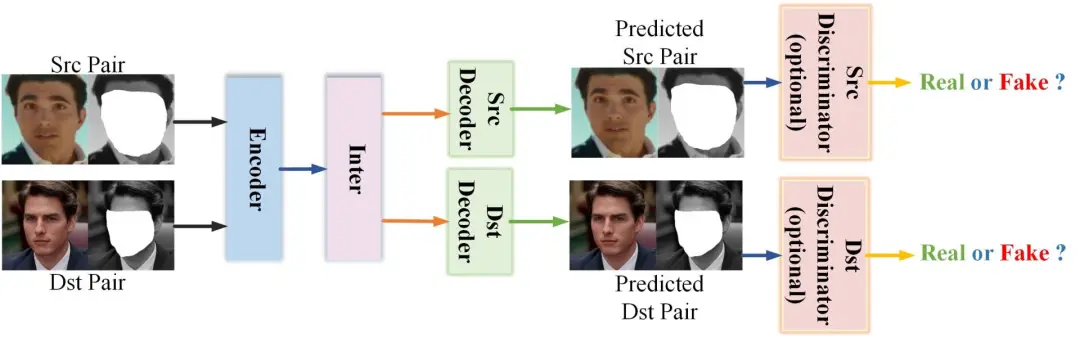
LIAE structure
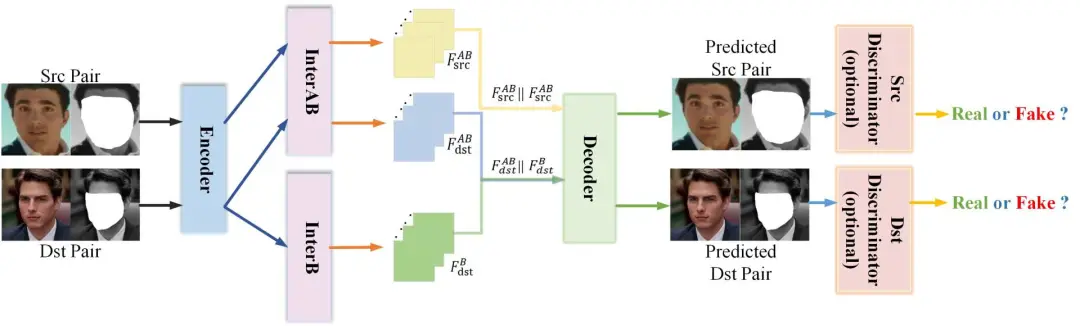
The DF structure consists of an encoder and an Inter with shared weights between src and dst, two decoders belonging to src and dst respectively.
The generalization of src and dst is achieved by the shared Encoder and Inter.
The DF structure can perform the task of face swapping but does not inherit enough information from dst, while the LIAE structure can be used to solve the consistency problem of light.
The LIAE structure is more complex, with an encoder that shares weights, a decoder and two separate inputs.
In addition, Lab uses hybrid loss (DSSIM+MSE) by default. dSSIM can generate faces faster, while MSE can provide better sharpness.
In addition, the authors use a real face mode, TrueFace, which can make the generated faces have better similarity with dst in the conversion stage.
From the results, there is a significant improvement in the quality of the final generated faces.
Part 3 Face Exchange
Previous methods often ignore the importance of the transformation phase.
Lab allows users to swap src faces to dst and reverse the process.

In order to maintain consistent face color, Lab provides five more color transfer algorithms (Reinhard color transfer, iterative distribution transfer, etc.).
Lab’s liae architecture model comes with light and shadow learning, so when dealing with different skin tones, face shapes and lighting conditions, the combination of the two faces will not look abrupt as long as the edges are feathered.
Finally, the face is sharpened.
Since SOTA models produce faces that are more or less smooth and lacking in minute details (e.g., moles, wrinkles), Lab integrates a pre-trained sharpening tool to sharpen faces.
Therefore, Lab integrates a pre-trained face super-resolution neural network for sharpening the blended faces.
Comparison of results
The authors used an open source project from the FaceForensics++ dataset to test the face swapping results.
Examples of face swapping with different expressions and face shapes
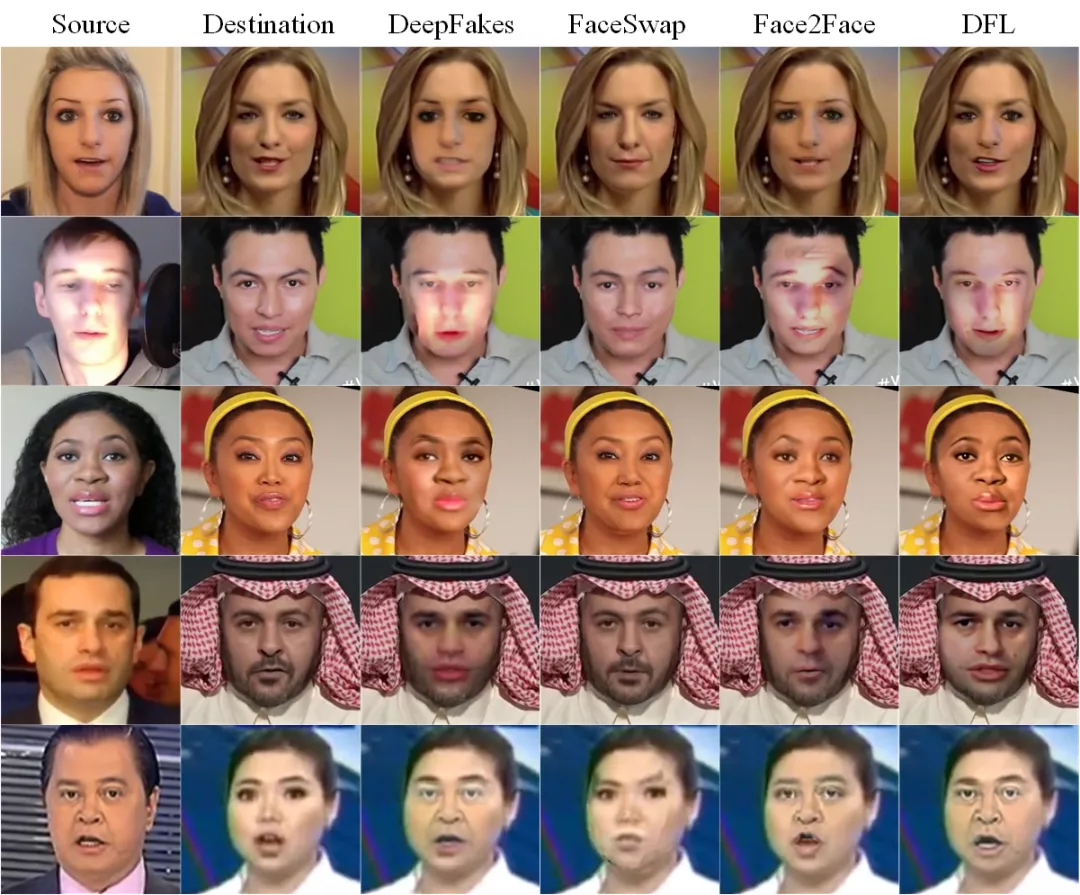
To be fair, the authors limited the training time to 3 hours and used a lightweight model with a DF structure: Quick96, which has an output resolution of 96×96.
In addition, the authors optimized the model using the Adam optimizer (lr=0.00005, β1=0.5, β2=0.999).
The models are trained on NVIDIA GeForce GTX 1080Ti GPUs and Intel Core i7-8700 CPUs.
FaceForensics++ Qualitative face replacement results for face images
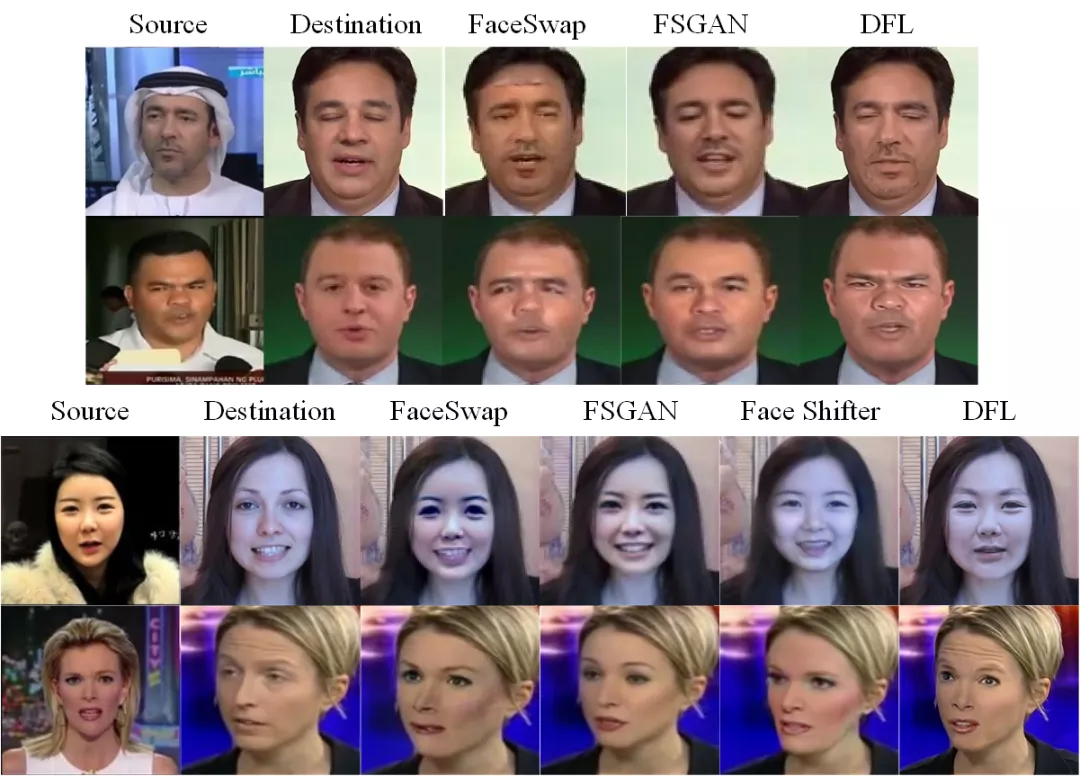
Lab can preserve more poses and expressions than DeepFakes and Nirkin’s models.

In addition, with the addition of the super-resolution network in the transformation stage, Lab can output more soulful eyes and well-defined teeth.
However, this effect cannot be reflected in the SSIM score.
Real-time face replacement, is it good or bad?
As researchers continue to pursue natural effects, AI face-swapping technology is becoming more and more “impeccable”.
Now the live-streaming industry is riding on the wings of technological development, allowing businesses to profit more.
The developer of the software, “Rolling Stone, who wishes to remain anonymous,” says that if the live-streaming industry can use this face-swapping software, the hosts with interesting souls but not enough faces can have high faces and improve the attractiveness of their live-streaming rooms.
It greatly reduces the cost of opening a live room for businesses, while also improving the attractiveness of the live room.
The developer also expressed his concern: once the software is widely used, people with bad intentions may use real-time face-swapping technology to commit fraud and blackmail.
Previous face-swapping technology can only change the face of the video at most, if you want to fraud, as long as you remain vigilant, the video will soon be revealed.
But if real-time face-swapping technology is used for fraud, in this virtual world of real and fake, there may be no way for most people to tell if the end of the screen is the “real one”.
If the scam is for older people like our parents, who can interact with them, they may easily be tricked into transferring money when their discernment is not high.
In this AI world, can we still keep the last sincerity between people?
Reference:
DeepFaceLive project address: https://github.com/iperov/DeepFaceLive
DeepFaceLab project address: https://github.com/iperov/DeepFaceLab
DeepFaceLab paper address: https://arxiv.org/pdf/2005.05535.pdf